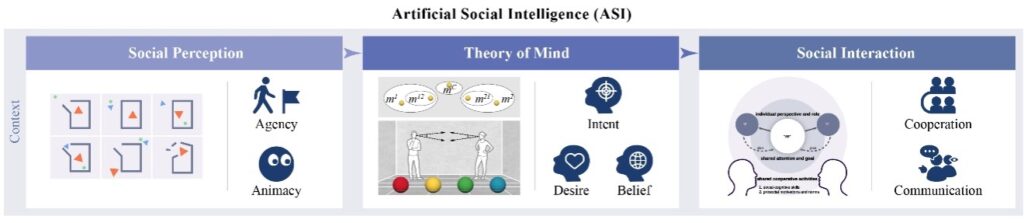
In recent years, considerable focus has been placed on the topic of general artificial intelligence, leading many to believe that the realization of such advanced intelligence is within grasp. Nevertheless, despite these advancements, numerous unresolved issues persist, with one prominent challenge being the development of Artificial Social Intelligence (ASI). In addition to comprehending the physical world, humans possess a remarkable level of social intelligence—an intellectual capacity encompassing the perception of social events, inference of others’ goals and intentions, and facilitation of social interactions. This exceptional social intelligence serves as a critical differentiating factor between humans and their closest primate counterparts. Nonetheless, harnessing this unique form of intelligence for artificial intelligence purposes presents an exceptionally arduous undertaking.
Published in CAAI, the review paper entitled “Artificial Social Intelligence: A Comparative and Holistic View” calls for attention to the field of Artificial Social Intelligence (ASI). The review delves into an in-depth study of the cognitive mechanisms behind social intelligence and explores how these mechanisms can be applied to the field of artificial intelligence to create more intelligent and human-like machines, thereby advancing towards general artificial intelligence.
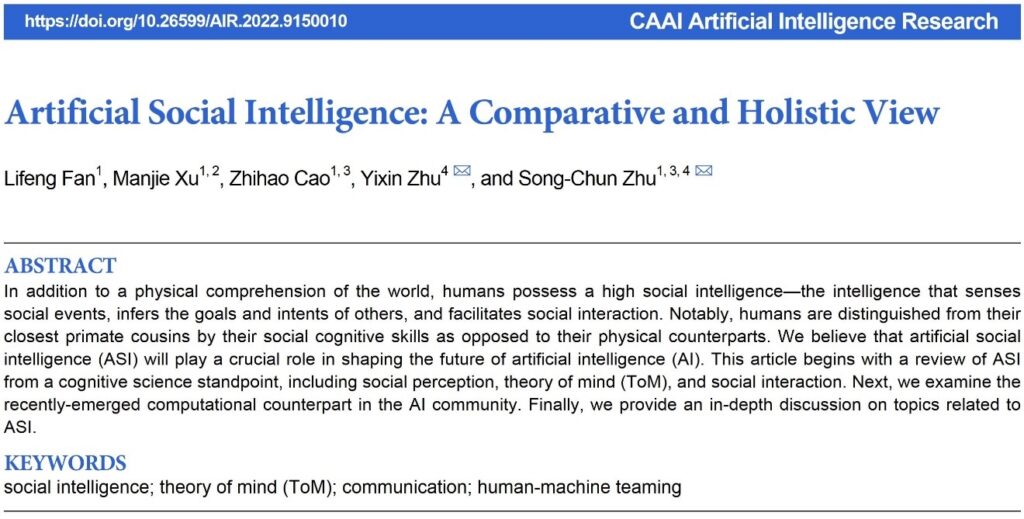
This paper presents a comprehensive exploration of social intelligence, delving into the unique cognitive abilities that underlie human social interactions and their application in the field of artificial intelligence. Specifically, the review adopts a dual approach, examining artificial social intelligence through the lenses of cognitive science and computational modeling. It encompasses subdomains such as social perception, Theory of Mind (ToM), and social interaction. The paper also discusses the significant challenges encountered in studying artificial social intelligence, available research resources within the field, and future development trends.
Social intelligence encompasses three interrelated aspects: social perception, Theory of Mind, and social interaction.
Social perception refers to humans’ capacity to perceive social attributes (such as animacy and agency) of stimuli in their environment. A crucial factor influencing social perception is dynamic structure, wherein complex social information can be inferred from the motion of simple geometric shapes. Social perception lies at the intersection of human perception and cognitive processing. By transforming basic signal stimuli into causal, living, and even intentional entities, social perception contributes to higher-level cognitive reasoning processes.
Theory of Mind (ToM) entails the understanding of one’s own and others’ mental states, including emotions, beliefs, intentions, and desires. It involves recognizing that individuals may possess different perspectives and cognitive processes. Initially studied in psychology and cognitive science, ToM has expanded its purview to the realm of artificial intelligence.
ToM holds particular significance in multi-agent and human-computer interaction settings, as each intelligent agent must comprehend the cognitive states of others to engage in effective interaction and accomplish cooperative tasks. A well-developed Theory of Mind enables intelligent agents to interpret and predict the intentions and behaviors of other agents, leading to more fruitful and successful collaborations.
The classic Sally-Anne test serves as a fundamental experiment in Theory of Mind, examining how children, during early cognitive development, comprehend others’ beliefs and false beliefs. It represents a pivotal milestone in children’s cognitive development according to developmental psychology research.
During infancy, infants exhibit gaze tracking behavior, recognizing themselves and others as conscious agents capable of intentional actions. Their perception of the world is shaped by their subjective experiences. At around 14 months, infants begin to gather visual information through movement and use eye gaze to confirm shared perceptual environments with others and themselves. Around 14 to 18 months, infants start grasping cognitive states such as desires, intentions, and emotions through eye direction.
By the ages of 3 to 4 years, children develop an understanding of the differences in beliefs and knowledge between themselves and others, thus comprehending false beliefs. This ability fully stabilizes around 5 to 6 years of age. In later stages of development, children establish a second-order Theory of Mind, involving the ability to predict one person’s thoughts or feelings about another person. This requires more complex cognitive processes.
While various methods, such as behavioral analysis, neuroimaging, and analysis of neural signals, are available to study Theory of Mind, there is a pressing need for a comprehensive understanding of what Theory of Mind entails, how humans utilize it, and the contributions of the neural system to its functionality.
Social interaction stands as a cornerstone of human social intelligence. When investigating social interaction, attention must be given to social cues, phenomena, rules, and mechanisms that facilitate more intricate and human-like communication and collaboration abilities in artificial social intelligence. Social cues, in particular, hold great importance within social interaction. Some noteworthy forms of social interaction encompass gaze communication (conveying information through eye contact), joint attention (multiple individuals attending to the same object or event), pointing (indicating a target or location using hands or other body parts), and cooperation (multiple intelligent agents working collectively to achieve a shared goal or task).
The review underscores the formidable challenges associated with Artificial Social Intelligence (ASI) in comparison to physical intelligence, with one prominent obstacle being its pronounced dependence on context. The notion of context encompasses various factors, ranging from the cultural background and general knowledge of a given population to the shared experiences between individuals. To illustrate this point, consider the following example: Suppose two friends, A and B, observe a bicycle parked outside a library. The interpretation of A’s intention behind referencing the bicycle hinges upon the contextual framework formed by their individual cognitive beliefs and shared beliefs. If both individuals are aware that the bicycle belongs to B’s boyfriend, C, then A’s intention may involve entering the library to locate C. However, if both individuals are aware of B and C’s recent breakup, A’s intention may revolve around suggesting not to go to the library.
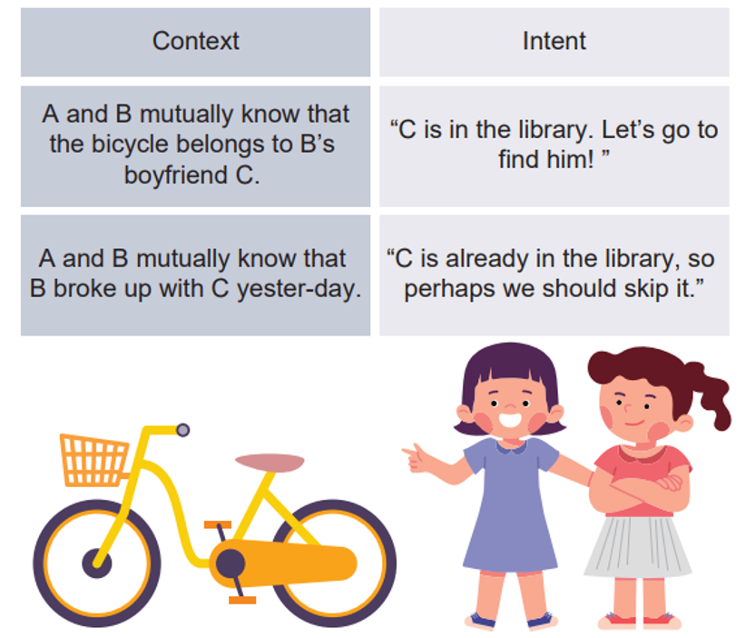
The real-world environment presents a multitude of challenges for addressing problems related to Artificial Social Intelligence (ASI). Its inherent complexity, ambiguity, and dynamic nature, coupled with partial observability and interactions among multiple intelligent agents, render conventional algorithms ill-equipped to tackle ASI effectively. Consequently, achieving ASI necessitates a comprehensive and holistic approach, as localized improvements often fail to yield desired overall performance enhancements.
Multidisciplinary research offers invaluable insights and inspiration in the pursuit of realizing Artificial Social Intelligence (ASI). A thorough exploration of human social intelligence enables a deeper comprehension of the fundamental principles, frameworks, reference systems, and testing benchmarks necessary for developing ASI with human-like attributes.
The review further suggests that the most promising approach to researching and developing Artificial Social Intelligence (ASI) lies in faithfully and systematically emulating the interactive patterns observed in human-human and human-environment interactions. Models should possess the ability to learn in diverse ways, akin to humans, encompassing lifelong learning, multitask learning, one-shot/few-shot learning, meta-learning, and other similar paradigms. This necessitates the creation of open and interactive environments, as well as thoughtful considerations on how to better incorporate human-like biases into ASI models.
In conclusion, the review highlights that despite remarkable advancements in artificial intelligence research, achieving human-level intelligence remains an ambitious goal. Artificial Social Intelligence (ASI) stands out as a crucial challenge on the path to realizing general artificial intelligence. It stresses the importance of treating ASI as an independent research field and urges AI researchers to engage in discussions and investigations pertaining to the intricate problems within this domain. This encompasses defining new problem domains, developing novel environments and datasets, establishing innovative evaluation methodologies, and devising cutting-edge computational models. Such endeavors hold the potential to catalyze groundbreaking advancements in both theory and computation, ultimately empowering artificial intelligence with high-level social intelligence and leveraging ASI to enhance human well-being.
Authors:
Lifeng Fan1, Manjie Xu1,2, Zhihao Cao1,3, Yixin Zhu4(✉️), Song-Chun Zhu1,3,4(✉️)
1 National Key Laboratory of General Artificial Intelligence, Beijing Institute for General Artificial Intelligence (BIGAI), Beijing 100080, China
2 School of Computer Science and Technology, Beijing Institute of Technology, Beijing 100081, China
3 Department of Automation, Tsinghua University, Beijing 100084, China
4 Institute for Artificial Intelligence, Peking University, Beijing 100871, China